
AML and CTF requires action to mitigate the risks, actually to disrupt and prevent money laundering. This lesson on AML/CTF investigations examines the analysis of geospatial and financial information to produce location intelligence.
This is the intersection of geospatial data of all types, to include social data, remote sensing where applicable, data analytics, and data visualization. Geospatial data sources enrich the initial available information for analysis from structured and unstructured data, open source, third-party, internal, and collected data. Think of how funds are transferred in the 21st century, the vast information accessible to evaluate risk summaries on FI, sectors, and countries. The role of the geospatial analyst is not as investigator or determiner of illegal activities, but tasked to analyze geospatial factors surrounding suspicious activity.
Combining a geospatial analytics methodology and tradecraft to enhance AML/CTF investigations, following a method, process, and secret approach to produce location intelligence. Analysts perform geospatial analysis to assess threats and hazards, discover and model patterns, relationships and networks, and apply predictive and prescriptive analysis to map likely areas at similar risks. These are key elements to risk assessments; however, the criminal intent goes beyond certain nations with bad or weak policies that allow opportunities for money laundering.
Laws, Higher Authority, and Official Organizations
Authorities from the local to global level direct effort and establish boundaries on the geospatial analyst's involvement in AML/CTF investigations to comply with procedures, protect client and business rights, and produce location intelligence of suspicious cases.
U.S. Laws governing banking and the financial sector.
- Bank Secrecy Act of 1970
- Money Laundering Control Act (1986)
- Uniting and Strengthening America by Providing Appropriate Tools Required to Intercept and Obstruct Terrorism Act of 2001 (USA PATRIOT Act) justice.gov/archive/ll/highlights.htm
- Intelligence Reform & Terrorism Prevention Act of 2004 govinfo.gov/content/pkg/PLAW-108publ458/pdf/PLAW-108publ1458.pdf
Higher Authorities publish expert guidance, conduct oversight, and provide support for AML/CTF investigations. A significant international organization for law enforcement and finance is the Financial Action Task Force (FATF) providing AML recommendations with a view to global risks to regulate financial sector, curb corruption, and assessments of specific country strengths and weaknesses.
- Financial Action Task Force (FATF) http://www.fatf-gafi.org
- US Treasury, Financial Crimes Enforcement Network ustreas.gov/fincen
- US Department of Statestate.gov
- US Secret Service secretservice.gov/ntac
- INTERPOL; countering financial crimes, payment card fraud, money laundering, and currency counterfeiting
- United Nations Office on Drugs and Crime (UNODC), The Money-Laundering Cycle (2019)
- World Bank, World Governance Indicator (WGI)
Process of geospatial analysis to produce Location Intelligence
For this lesson and case study, you will examine a sequence of geospatial analysis methods and pertinent AML/CTF analytic questions. This involves complex geospatial analysis to detect patterns, corridors, and networks. Examine the process, purpose, and value of descriptive, real time, predictive, and prescriptive analysis techniques. Similar methods include fraud ring analysis, link analysis, or network analysis.
Investigators ask investigative, analytic, behavioral, and risk questions. We interpret the question to determine a method of geospatial analysis to expose:
- the flow of illicit funds,
- funding streams, and
- the potential risks to nearby FIs.
The workflow depends on the business question an analyst is addressing. Bank and FI objectives range in scope, possibly to identify money laundering, follow up on a client's SAR, prevent money laundering, and comply with laws, financial sector policies, or local adverse situations. Each situation requires an approved target and organized method.
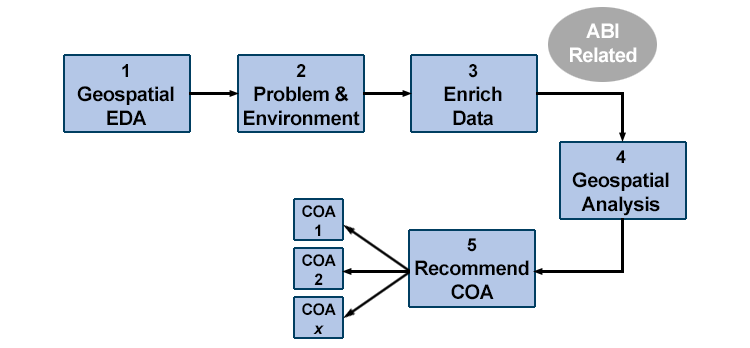
The foundation of geospatial analysis in AML/CTF is a workflow, as depicted in Figure 6.1, a process to:
- Identify suspicious activity or potential of illicit activity. This step often starts with exploratory data analysis (EDA) using descriptive and graphical statistical tools to explore and understand each dataset.
- Restate the problem as a question with outcomes that support an investigation and define the environment.
- Assemble and enrich all relevant geospatial information, data, and imagery if applicable. This is similar to activity-based intelligence (ABI) through which human behaviors, events, and transactions are analyzed to produce pattern of life assessments. Analysts who produce ABI focus on events as recognizable movements or changes conducted by an entitiy and spatial metadata components, relating them to time and space.
- Perform geospatial analysis to assess threats and hazards, model patterns, relationships, and networks. To optimize the location intelligence assessment, analysts may apply prescriptive analytics forming valid courses of action for decision makers.
- Present Recommendations, Courses of Action. Map, visualize, chart, and summarize for presentation or to develop analytic recommendations to alert authorities.
To that extent, this is not simply a Boolean analysis to layer all our banking and law enforcement data sets to locate hubs of money laundering. It's useful to use domain-specific theories to better understand the actual phenomena being investigated.
It also requires choosing the appropriate method or technique of geospatial analysis to describe the situation and answer key questions.
Analysis technique | analytic question | value to aml/ctf investigation |
---|---|---|
Descriptive | What happened? | Proven investigative technique to build elements of a case. |
Diagnostic | What's happening? | Gaining situational understanding of AML/CTF activity and locations. |
Predictive | What could happen? | Likelihood of similar actions occurring in a different location. |
Prescriptive | What should we do? | Recommending courses of action for decision. |
Prescriptive analytics adds a previously unappreciated decision advantage to AML and CTF investigations for the business, financial, and public safety sectors. Where appropriate and within the scope of duties, recommended courses of action provide location intelligence insights. Optimization and simulation algorithms advise on possible outcomes that calculate predictions, providing courses of action to decision makers.
In geospatial analytics, coding and AI may automate routine tasks and elevate alerts, reports, and situational awareness to well-trained analysts. Advanced machine learning is currently applied to identify high-risk procedural aberrations, false links, and patterns of fraud. Location intelligence provides insights of regions, customers, and FIs where multiple-SAR related transactions meet regulations or cumulative illicit activity falls below thresholds.
Case Study
Identify regions likely exposed to:
- The flow of illicit funds
- Potential funding streams
- Risks to nearby FI’s
Recognize the Global Financial Audit Trail, "Follow the Money" to understand the broader global financial networks and investment strategies. Bad money is intermingled with good money in a complex attempt by criminals to throw off investigators and complicate a financial audit trail.
By leveraging the wealth of data available to FIs and exploring additional physical features, such as important rail and road networks, location intelligence can be utilized to enhance the understanding of potential illicit funding corridors. There's a process for geospatial analysts to work, which you may consider for the case study.
- Integrate business segments to detect banking anomalies regarding customers, FIs, and transactions
- Discover patterns of money laundering
- Assist FI’s to connect additional geospatial information to “know their customers”
- National and International practices to enrich data for discovery
- Create models to identify and visualize patterns, risk areas, corridors
Presentations
Present findings for actionable decision.
Present location intelligence for referral; summary, charts, maps, pattern diagrams
Submit report to authorities
It's important to recognize the significance of visualizations for decision-making; seeing patterns emerge from data, identifying linked networks, and discovering instances of suspicious activity.
Advanced Analytics
Choosing the right tool falls into the analyst's ability for critical thinking. This encompasses math, statistics, computer science, and analytical reasoning. One will use a variety of geospatial analytical tools; but the software platform doesn't drive analysis. In your studies, you are learning methods of geospatial analysis, developing elements of tradecraft, and applying location intelligence workflows to a problem. Learning to use a particular software or tool is more training than education. Develop your critical thinking through your courses and personal continuing education.
The AML/CTF case study is a learning exercise to reinforce the main points of location intelligence and material in Lessons 5 & 6. In this section, we're presenting advanced geospatial analytics material, which may require additional software tools to accomplish (outside this course and not for the assignments). This may be a refresher from other experiences or your introduction to advanced visualizing methodologies:
1. Outliers or anomalies
Spatial outliers and anomalies are spatial objects whose non-spatial attributes differ from their spatial neighbors. This technique is quite effective with suspicious behavior and outlier transaction detection. May be represented by a graph analysis of events by latitude and longitude to identify outliers; and then dive deeper into feature attributes to detect anomalies and refer for other investigative tools or methods.
2. Colocation patterns
Colocation patterns can be detected through event-centric models to build neighbor groupings of data points. Alteryx (Alteryx) is an example of a data management software in which the analyst creates a workflow to statistically analyze and filter structured and unstructured financial data. A robust analytic software tool supports exploring a wide range of datasets to uncover patterns. The advantage in point data analysis is to apply statistical significance tests to remove chance patterns. Data management and data analytics tools, e.g. R, GeoDa, SQL, predictive analytics or graph analytics, can perform colocation pattern detection where geolocated events are often sited in a geographic neighborhood.
3. Hotspot detection
Geospatial hot spot regions are significantly more active than surrounding areas in density, frequency, and appearance. This method is common with ABI and fraud ring analysis. Signature Analyst (Maxar) has been successfully applied to depict the likelihood of terrorist threats and event corridors in foreign conflicted areas.
4. Teleconnection detection in space & time
Teleconnection technique is used to discover pairs of correlated spatial time series at large distances, with either positive or negative correlation. There are situations and conditions where imagery adds value in AML/CTF investigations to detect, measure, and visualize patterns in space and time. Imagery is remotely sensed from space, airplanes, unmanned aerial systems, and surveillance video/photos. Tools like ENVI (L3/Harris Geospatial) provide reliable automation of complex and repetitive digital functions.
5. Predictions
Predictive analysis works best with a Bayesian approach, calculating probabilities on georeferenced information from numerous datasets. Spatial prediction relies on selection of an appropriate model for classification and regression, relying on accurate training samples. Signature Analyst (Maxar) effectively works through dependent and independent variable relationships to identify likely locations where similar AML/CTF events may occur.
The financial community recognizes the advantages of combining location intelligence workflows with advanced computing applications. Current literature provides insight on companies researching and programming AI to automate routine foundational tasks, e.g. elevate alerts, reports, situational awareness to analysts. Advanced machine learning has advantages to cue patterns, financial anomalies, and - since we're dealing with the human domain - to rule out false leads.
Errors, Cautions, False Leads
Geospatial scientists understand the potential errors which traditional data mining creates on geospatial data. You may have found that traditional density-based clustering methods will generally output chance patterns if applied to geospatial hotspot detection. Methods that test for statistical significance can potentially reduce such chance patterns.
Unlike classic data mining for businesses, these geospatial techniques must handle spatial point distributions and the associated challenges of auto-correlation.
False positives or false leads present challenges in AML/CTF investigations. Data or events may appear suspicious when they are actually the result of standard transactional activity. This may be caused by randomness in data, errors of bias in prediction assumptions, AI training data, or investigation. People are not always predictable. The purchase of a $9,000 necklace isn't always to hide a transaction below the $10,000 bank reporting threshold. There's significant corporate marketing that goes into selling jewelry, and one buyer may be inspired to spend less than $10,000 on a fancy necklace.
Conclusion
What actions should a financial organization take in finding, freezing, and forfeiting criminally derived income and assets?
All of this analysis effort is to support the people involved:
- Customers
- Analysts
- Bank tellers trained to spot odd behavior, and
- Senior decision makers
Analysts detect patterns, quantify the events, risks, and potential consequences. Investigators and decision makers follow these leads to stop criminal and terrorist access to money.
Developing new prescriptive methods, deploying machine learning, AI, and critical thinking will advance the discipline of investigating and stopping financial criminal activity.
Proven geospatial analytic methods of location intelligence transform the efficiency of investigations, location-based business problem-solving, and global commerce which is moving at high speed, undergoing great change.
Skim:
- U.S. Securities and Exchange Commission. 2018. Anti-Money Laundering (AML) Source Tool for Broker-Dealers. 4 Oct 2018. Online.
- U.S. Treasury. 2005. U.S. Money Laundering Threat Assessment.
- Bureau of Justice Assistance. 2019. The State and Local Anti-Terrorism Training (SLATT) Program. U.S. Department of Justice.