Read...
A famous Penn State meteorology professor, long since retired, had a famous saying with regard to model output. “All that I can be sure of,” he’d say, pointing at the forecast map, “is that the weather is not going to look anything like that.” Was this the reaction of a curmudgeon, a disbeliever in “new-fangled” weather forecasting technology? Or was there more to his statement than simple disbelief?
Early in my career, numerical weather models were just gaining steam and their flaws were quite obvious, even in the short term. Still, it was difficult not to latch on to the “model of the day” and run with what it had to say. Today, I think that the temptation to blindly believe what the models are telling us is even greater. Why is that? Well, you’ve probably realized by now that there is an avalanche of model data available to even the casual user. Models that produce output every hour and at a resolution of just a few kilometers surely must be accurate, aren’t they? Plus, when displayed properly, this data looks awesome. Some precipitation output looks “just like” live radar data. That must mean it’s for real, yes?
What about all the arguments about who has the better model? Is the “Euro” better than the “GFS” at 60-72 hours? Have you perhaps heard someone argue that the “NAM is really the model to believe for a particular developing storm system?” The temptation to bet on a model as you would a racehorse is great, but I strongly caution against it. While models can provide general guidance in any one instance, relying too heavily on any particular model solution has no basis in science whatsoever and WILL get you in trouble more times than not.
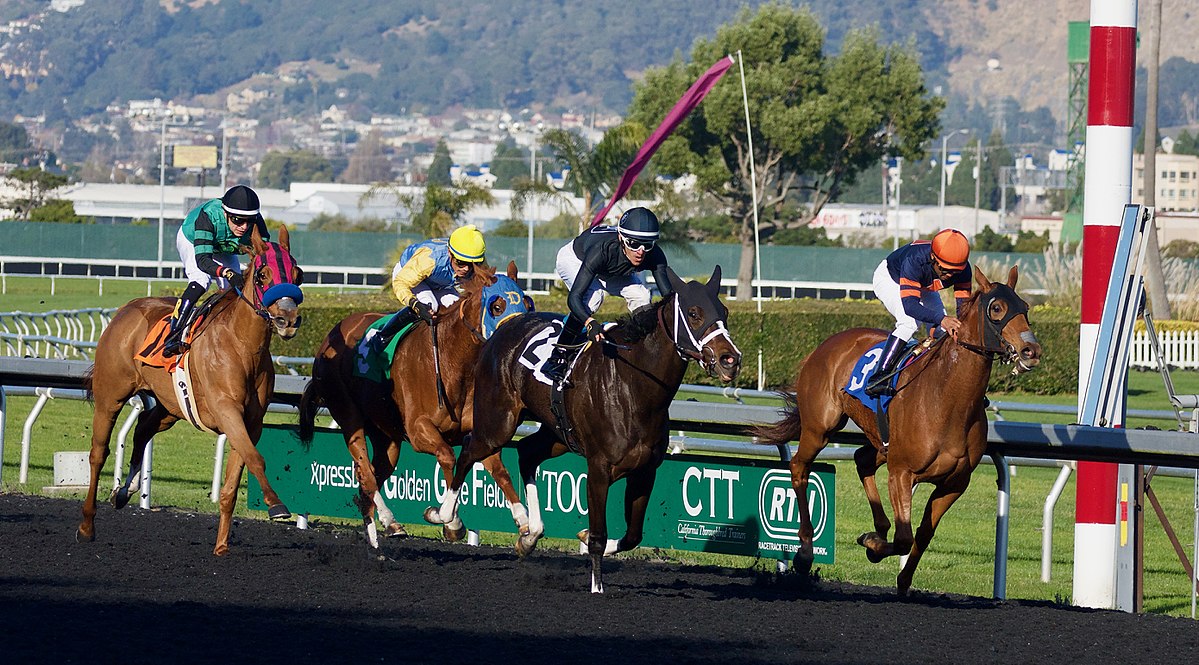
All is not lost, however. Because you have access to all of the raw data, you can use model output (along with some numerical wrangling) to shape your final decision-making process. Model Output Statistics (MOS) are a good example. In a nutshell, MOS runs the output of a dynamical model through a multi-variable regression algorithm in order to better predict surface variables. Believe it or not, dynamical models perform as poor predictors of surface variables. This is because surface processes are extremely complex, and dynamical models often parameterize these processes. However, if model predictions are compared with observations over a long period of time, certain patterns emerge that allow for a better prediction of surface variables.
So, what’s the takeaway? Model data IS a valuable resource… if used properly. In addition to peering into the future, the small resolution of numerical models makes for an ideal way to “fill in” observational gaps, as long as techniques are used to marry numerical and observational data. In later courses, you will learn such techniques. But for now, know that numerical model data should be used with care.