
One of the main advantages of digital data is that they can be readily processed using digital computers. Over the next few pages, we focus on digital image processing techniques used to correct, enhance, and classify digital, remotely sensed image data.
7.4.1 Image Correction
As suggested earlier, scanning the Earth's surface from space is like scanning a paper document with a desktop scanner, only a lot more complicated. Raw remotely sensed image data are full of geometric and radiometric flaws caused by the curved shape of the Earth, the imperfectly transparent atmosphere, daily and seasonal variations in the amount of solar radiation received at the surface, and imperfections in scanning instruments, among other things. Understandably, most users of remotely sensed image data are not satisfied with the raw data transmitted from satellites to ground stations. Most prefer preprocessed data from which these flaws have been removed.
Relief displacement is one source of geometric distortion in digital image data, although it is less of a factor in satellite remote sensing than it is in aerial imaging, because satellites fly at much higher altitudes than airplanes. Another source of geometric distortions is the Earth itself, whose curvature and eastward spinning motion are more evident from space than at lower altitudes.
The Earth rotates on its axis from west to east. At the same time, remote sensing satellites like IKONOS, Landsat, and the NOAA satellites that carry the AVHRR sensor, orbit the Earth from pole to pole. If you were to plot on a cylindrical projection the flight path that a polar orbiting satellite traces over a 24-hour period, you would see a series of S-shaped waves. As a remote sensing satellite follows its orbital path over the spinning globe, each scan row begins at a position slightly west of the row that preceded it. In the raw scanned data, however, the first pixel in each row appears to be aligned with the other initial pixels. To properly georeference the pixels in a remotely sensed image, pixels must be shifted slightly to the west in each successive row. This is why processed scenes are shaped like skewed parallelograms when plotted in geographic or plane projections.
The reflectance at a given wavelength of an object measured by a remote sensing instrument varies in response to several factors, including the illumination of the object, its reflectivity, and the transmissivity of the atmosphere. Furthermore, the response of a given sensor may degrade over time. With these factors in mind, it should not be surprising that an object scanned at different times of the day or year will exhibit different radiometric characteristics. Such differences can be advantageous at times, but they can also pose problems for image analysts who want to create a mosaic, by adjoining neighboring images together, or to detect meaningful changes in land use and land cover over time. To cope with such problems, analysts have developed numerous radiometric correction techniques, including Earth-sun distance corrections, sun elevation corrections, and corrections for atmospheric haze.
To compensate for the different amounts of illumination of scenes captured at different times of day, or at different latitudes or seasons, image analysts may divide values measured in one band by values in another band, or they may apply mathematical functions that normalize reflectance values. Such functions are determined by the distance between the earth and the sun and the altitude of the sun above the horizon at a given location, time of day, and time of year. To make the corrections, analysts depend on metadata that includes the location, date, and time at which a particular scene was captured.
In addition to radiometric correction, there is a need for images to be geometrically corrected. Geometric correction and orthorectification are two methods for converting imagery into geographically-accurate information. Geometric correction is applied to satellite imagery to remove terrain related distortion and earth movement based on a limited set of information. In contrast, orthorectification uses precise sensor information, orbital parameters, ground control points, and elevation to precisely align the image to a surface model or datum. At the end of this chapter, you will read more about orthorectification as it relates to aerial imagery.
7.4.2 Image Enhancement
Correction techniques are routinely used to resolve geometric, radiometric, and other problems found in raw remotely sensed data. Another family of image processing techniques is used to make image data easier to interpret. These so-called image enhancement techniques include contrast stretching, edge enhancement, and deriving new data by calculating differences, ratios, or other quantities from reflectance values in two or more bands, among many others. This section considers briefly two common enhancement techniques: contrast stretching and derived data. Later, you'll learn how vegetation indices derived from two bands of AVHRR imagery are used to monitor vegetation growth at a global scale.
Consider the pair of images shown side by side below. Although both were produced from the same Landsat MSS data, you will notice that the image on the left is considerably dimmer than the one on the right. The difference is a result of contrast stretching. As you recall, Landsat data have a precision of 8 bits, that is, reflectance values are encoded as 256 intensity levels. As is often the case, reflectance in the near-infrared band of the scene partially shown below include an intensity range of only 30 to 80 in the raw image data. This limited range results in an image that lacks contrast and, consequently, appears dim. The image on the right shows the effect of stretching the range of reflectance values in the near-infrared band from 30-80 to 0-255, and then similarly stretching the visible green and visible red bands. As you can see, the contrast-stretched image is brighter and clearer.
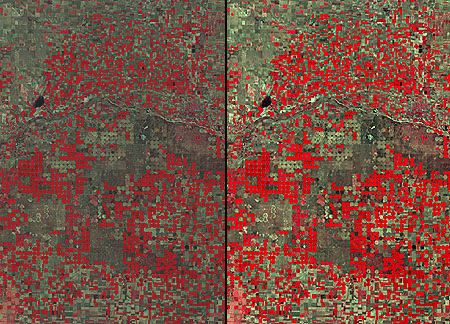
7.4.3 Image Classification
Along with military surveillance and weather forecasting, a common use of remotely sensed image data is to monitor land cover and to inform land use planning. The term land cover refers to the kinds of vegetation that blanket the earth's surface, or the kinds of materials that form the surface where vegetation is absent. Land use, by contrast, refers to the functional roles that the land plays in human economic activities (Campbell, 1983).
Both land use and land cover are specified in terms of generalized categories. For instance, an early classification system adopted by a World Land Use Commission in 1949 consisted of nine primary categories, including settlements and associated non-agricultural lands, horticulture, tree and other perennial crops, cropland, improved permanent pasture, unimproved grazing land, woodlands, swamps and marshes, and unproductive land. Prior to the era of digital image processing, specially trained personnel drew land use maps by visually interpreting the shape, size, pattern, tone, texture, and shadows cast by features shown in aerial photographs. As you might imagine, this was an expensive, time-consuming process. It's not surprising then that the Commission appointed in 1949 failed in its attempt to produce a detailed global land use map.
Part of the appeal of digital image processing is the potential to automate land use and land cover mapping. To realize this potential, image analysts have developed a family of image classification techniques that automatically sort pixels with similar multispectral reflectance values into clusters that, ideally, correspond to functional land use and land cover categories. Two general types of image classification techniques have been developed: supervised and unsupervised techniques.
7.4.3.1 Supervised Classification
Human image analysts play crucial roles in both supervised and unsupervised image classification procedures. In supervised classification, the analyst's role is to specify in advance the multispectral reflectance or, in the case of the thermal infrared band, emittance values typical of each land use or land cover class.
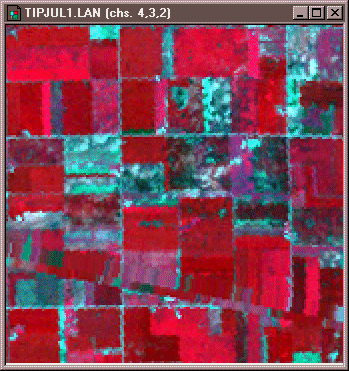
For instance, to perform a supervised classification of the Landsat Thematic Mapper (TM) data shown above into two land cover categories, Vegetation and Other, you would first delineate several training fields that are representative of each land cover class. The illustration below shows two training fields for each class; however, to achieve the most reliable classification possible, you would define as many as 100 or more training fields per class.
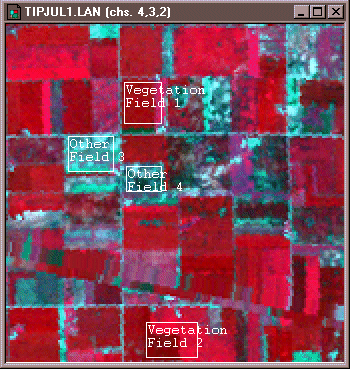
The training fields you defined consist of clusters of pixels with similar reflectance or emittance values. If you did a good job in supervising the training stage of the classification, each cluster would represent the range of spectral characteristics exhibited by its corresponding land cover class. Once the clusters are defined, you would apply a classification algorithm to sort the remaining pixels in the scene into the class with the most similar spectral characteristics. One of the most commonly used algorithms computes the statistical probability that each pixel belongs to each class. Pixels are then assigned to the class associated with the highest probability. Algorithms of this kind are known as maximum likelihood classification. The result is an image like the one shown below, in which every pixel has been assigned to one of two land cover classes, vegetation and “other.”
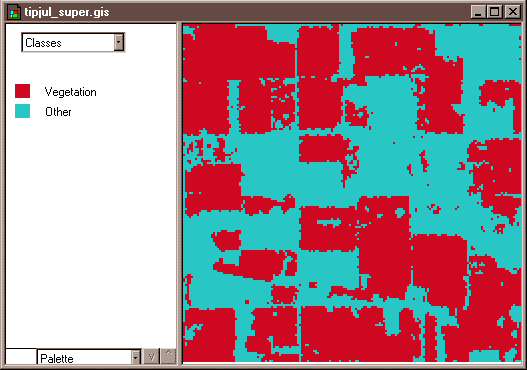
7.4.3.2 Unsupervised Classification
Image analysts play a different role in unsupervised classification. They do not define training fields for each land cover class in advance. Instead, they rely on one of a family of statistical clustering algorithms to sort pixels into distinct spectral classes. Analysts may or may not even specify the number of classes in advance. Their responsibility is to determine the correspondences between the spectral classes that the algorithm defines and the functional land use and land cover categories established by agencies like the U.S. Geological Survey. An example in Section 7.7 below outlines how unsupervised classification contributes to the creation of a high-resolution national land cover data set.
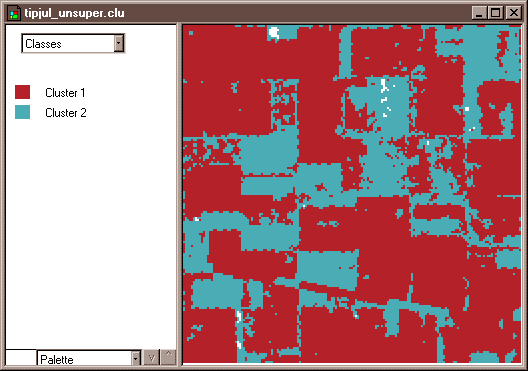
Practice Quiz
Registered Penn State students should return now take the self-assessment quiz about Image Processing.
You may take practice quizzes as many times as you wish. They are not scored and do not affect your grade in any way.