How Good are GCMs?
This is obviously a very important question — if we are to rely on these models to guide our decisions about the future, we need to have some confidence that the models are good. The most important approach is to see if the model can simulate the known climate history. We set the model up to represent the state of the climate at some point in the past — say 1900 — and then we see how well the model can reproduce what actually happened.
As you can see in the figure below, the models are collectively quite good:
In this figure, the black line is the instrumental global average temperature (as an anomaly, which is a departure from the mean value from 1901 to 1950), the yellow lines represent the output from 58 model runs by 14 different models, and the red is the average of those 58 runs. The vertical gray lines are times of major volcanic eruptions, which are always followed by a few years of cooler temperatures.
Now, let’s look at how closely the models can simulate the spatial pattern of temperatures over the Earth. To begin with, we’ll look at January temperatures for the time between 2003 and 2005 — here is what we can reconstruct from observations (which are better in some places than others — we know the Sea Surface Temperature (SST) much better than the land temperature, since SST is very precisely measured by satellites).
Now, we look at the same time period from a model simulation.
You can see that in general, they are quite similar to each other, but we can gain a bit more insight into the relationship between the observations and the model by subtracting the model from the observations — the result is this:
Here, you can see that the model and the observations are generally quite close, within a couple of degrees of zero, where zero would be a perfect match. Areas that are yellow to orange are regions where the actual temperature is greater than the modeled temperature; blue areas are regions where the actual temperature is lower than the model. It would be a challenging task to figure out the cause of these differences, but at a very fundamental level, it is related to the fact that things like clouds are very important to the climate system, and the processes that actually form clouds occur on such a small scale that the models cannot resolve them. Cloud formation is one example of what the modelers call a sub-grid process, and modelers have to devise clever ways of getting around this. This is an area where refinements continue to occur, but for the time being, we see that the models do an impressive job, but not a perfect job. As you can see in the above results, models tend to underestimate the temperatures on land, so we should consider model results for the future to also underestimate the true temperatures.
If we average over longer time periods and also average many model results together, we get what climate modelers refer to as an ensemble mean, and these ensemble means do a remarkably good job of matching the observations, as is shown below.
In this figure, the observed annual mean temperatures for the time period 1961-1990 are represented by the black contour lines, labeled in °C (-56°C in Antarctica and about 24°C in equatorial Africa). The colors represent the model temperatures (from 14 models, for the same 1961-1990 time period) minus the observations; positive values mean the models estimate temperatures that are too high. On the whole, the models slightly underestimate the temperatures, and they have particular problems at very high latitudes and in areas that are topographically complex.
As mentioned above, one of the important aspects of GCMs is that they calculate the precipitation, and this provides another means of evaluating how good the models are. The figure below shows a comparison of the observed annual precipitation and the average of the 14 models used by the IPCC study.
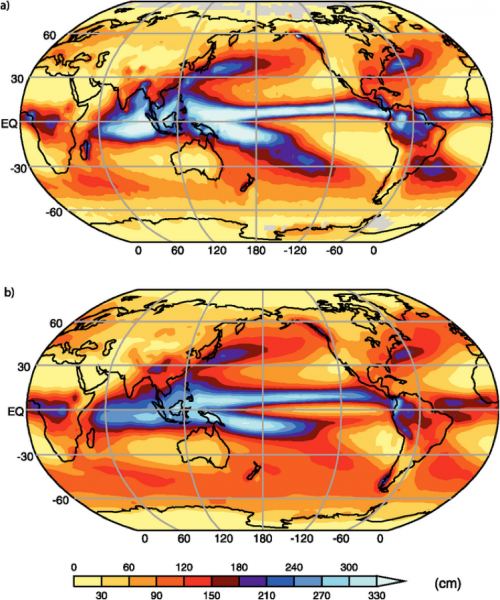
As can be seen, the models, on average, do quite well at simulating the global pattern of precipitation.
AOGCMs calculate the circulation and temperature of the world’s oceans, and we compare their ability in that regard by comparing the model-generated temperatures averaged over 1957 to 1990 with the observations from that time period.
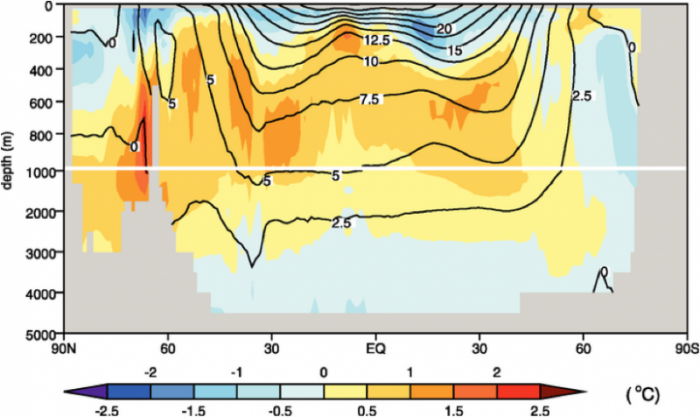
In this figure, we see the latitude-averaged observed ocean temperature from 1957 to 1990 in the contours, and the average model ocean temperatures minus the observed temperatures in colors. For most of the oceans, the models are ± 1°C from the observations.
In sum, it should be fairly clear that although these models are incredibly complicated, they do a fairly good job of reproducing the temporal and spatial characteristics of our climate, especially when we look at longer time averages. In other words, if we compare the model results for a given day with the actual observations of that day, the agreement is not very good; the agreement gets better on a monthly-averaged basis, and it gets even better on an annually-averaged basis. Today, models are in a constant state of improvement, with certain elements, such as the impact of clouds, needing a lot more understanding.